Authors: Judi Fusco, Jodi Asbell-Clarke, and Bernadette Sibuma
Printer-Friendly PDF | Google Doc for Comments | – Questions? Contact CIRCL.
Overview
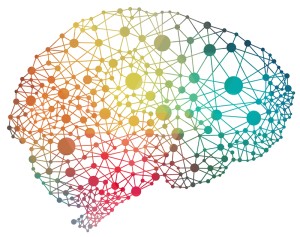
Cyberlearning researchers and neuroscientists are beginning to explore new methods to understand connections between classroom practice, neuroscience, and educational neuroscience. Together, they are investigating questions such as: How can neuroscience impact learning and teaching in the classroom? How can learning and teaching practices inform neuroscience models? What exciting opportunities and questions lie ahead at the convergence of neuroscience and educational research? What ethical and logistical considerations must we keep in mind while designing a research agenda in this area?
From neuroscience to cyberlearning may seem like a far reach. Why now? Advances in technology are enabling researchers to explore connections between neuroscience and learning science in new ways. For example, new methods enable the integration of multiple streams of data to build on multimodal models of learning, using electroencephalogram EEG and other physiological data gathered in classrooms (or labs). More portable and affordable neurological and physiological sensors now make it possible to do brain-based research outside of the lab in more authentic learning contexts, such as classrooms and gaming environments.
The convergence of neuroscience and cyberlearning could provide new insights into why particular interventions help some learners but not others. Perspectives from neuroscience can help refine our understanding about who is helped, how much they are helped, and under what conditions will the interventions may help. Cyberlearning has a key role to play in educational research, particularly as tools and methods enable feasible studies “in the wild” of classrooms and everyday activity. This primer discusses some of the key lessons and issues related to the convergence of neuroscience and educational research. In the next section, we provide a brief overview of neuroscience concepts.
Background Concepts
Generally, research on cognitive neuroscienceseeks to understand the relationships between brain structures and functions, such as perception, thinking, and learning. For example, cognitive neuroscience research can examine the role of the prefrontal cortex in executive functions and the hippocampus in memory formation. Findings in cognitive neuroscience research may converge with cognitive psychology research. Cognitive neuroscience and affective neurosciencer esearch also overlap in the investigation of the relationship between emotional and cognitive processes. Affective neuroscience considers the neural mechanisms involved in emotion. Initially, research on cognitive aspects of learning was separate from research on emotions, but recently neuroscience has shown how some of the same brain regions are involved in both emotional processing and cognitive processing. This relationship has implications for the design and development of learning environments.
In addition, the emerging area of educational neuroscience (sometimes called Mind, Brain, Education or MBE) links cognitive neuroscience with educational and learning theories. While some might consider educational neuroscience as a subset that overlaps different fields, others consider educational neuroscience a distinct collaborative attempt to build tools, methods, and frameworks across (human cognitive) neuroscience, (cognitive) psychology, and educational practice “without imposing a knowledge hierarchy” (Howard-Jones, et al., 2016). In the rest of this section we discuss methods, tools, and concepts that are foundational to neuroscience, cyberlearning, and/or to their convergence.
Techniques to Measure Neural Activity. Although direct measures of spatial neural activation and imaging of the brain using fMRI (functional Magnetic Resonance Imaging) or PET (Positron Emission Toography) are not practical in the classroom, there are portable physiological measures––such as fNIRS (functional near infrared spectroscopy), EEG (electroencephalogram), and eye-tracking––that can give an indication of where activity is occurring in the brain in real-world learning environments. Using such portable and less expensive tools, researchers have begun to examine the connections between brain activity and the process of learning in classrooms and everyday settings. In addition, researchers can bring students, teachers, or other types of learners to a lab so that neurophysiological recordings (fMRI or PET) can be collected before and then again after a classroom or other learning experience.
Attention and Executive Function. Attention and executive function are processes that impact how learners take in new information and build knowledge. Learners must attend to salient information, practice the coding of information repeatedly, and recode the information in a variety of contexts. Executive functions include a set of cognitive processes (including metacognition, self-regulation, as well as working memory, arousal, problem-solving, shifting activities, organizing, and self-monitoring) that are fundamental to learners’ ability to process and retain new information.
Attention has been argued to be a precursor to executive function, while others argue that executive function is an underlying ability of attention. Cognitive neuroscience studies have examined attention and executive function-related processes that affect performance. For example, brain research supports theories about how the stress or anxiety caused by stereotype threat can hurt performance by putting extra load on a person and reducing their working memory capacity in that situation (Hofmann, Schmeichel, & Baddeley, 2012; Rydell, McConnell, & Beilock, 2009; Mangels, et al., 2012); we also know that media multitasking negatively affects performance on a task (Moisala, et al., 2016).
Several different types of instructional activities have been designed to support attention and executive functions. Digital and non-digital games as well as curriculum interventions have been designed to focus on improving executive functions. In addition, many neurogames that include attention and executive function tasks inspired by neuroscience research are being examined to determine if they can serve as reliable measures of performance. Cyberlearning technologies could potentially link non-classroom types of interventions with classroom work to create new technologies and genres for learning. NSF has sponsored work on executive functions in educational neuroscience to better understand if multi-factor EF training through a novel-game based approach leads to better academic achievement, especially in math and reading.
Social-Emotional Connections. Cognitive, socio-emotional, and physiological process are part of all learning processes. Neuroscience has shown that cognitive functions depend on activity from areas of the brain traditionally thought of as “emotional” areas (e.g., amygdala and hypothalamus) thus emotional functions are actually part of systems traditionally thought of as cognitive (Pessoa, 2008). From neuropsychology, Damasio (1999) found that in patients suffering from brain damage to areas associated with emotional processing but who otherwise had cognitive processing areas intact, performance was compromised on cognitive tasks such as decision making and learning. In educational neuroscience, Immordino-Yang (2015) examines how emotional activation can help with learning. Lindquist and colleagues (2012) add evidence to the need for unifying the cognitive and emotional views and propose a more psychological constructionist (Lindquist and Barrett, 2008) approach to thinking about emotion. This and related research may have implications for thinking about affect and emotion in cyberlearning research.
Mirror neurons or mirror systems have been investigated as part of how we connect with others. The mirror system is a network of brain areas which process other people’s actions in a way that is similar to how our own actions are carried out. The mirror system may play a role in how we learn through observation, how people empathize with one another, and how language developed in our species (Rizzolatti, Fogassi, Gallese, 2006). Related research has shown that when we see an individual who we find likeable experiencing pain, we show an empathic response to his or her pain. However, if we see someone for whom we feel that the pain might be justified (e.g., as a punishment for unfair actions), fMRI scans show that we find satisfaction from their pain––men more so than women (Singer, Seymour, O’Doherty, Stephan, Dolan, & Frith, 2006). Research from psychology and neuroscience related to mirror systems, Theory of Mind, empathy, and social cognition might serve to inform the structuring of social interactions that could be relevant to cyberlearning researchers as they design learning environments. In addition, the activity of neural mirroring systems when researching observational learning (e.g., skill acquisition) should be considered.
The default mode network (DMN) of the brain (Raichle, 2015), or the “resting state” of the brain, may be important for understanding learning and learning environments (Howard-Jones, Jay, Mason, & Jones, 2015; Immordino-Yang, Christodoulou, & Singh, 2012). The DMN is typically not active during cognitive tasks, but is active during tasks involving episodic memory or understanding the self in relation to the work in “constructive internal reflection” (Immordino-Yang, 2016). Other research on the DMN shows that it overlaps with areas of the brain that are responsible for tasks related to social cognition (Mars, Neubert, Noonan, et al., 2012; Li, Mai, & Liu, 2014; Lieberman, 2013). Recent research shows that connectivity of the DMN to the prefrontal cortex can be affected by trauma and poverty (Weissman, et al., 2018). The functional role of the DMN is still being determined; it may play a role to help with automatic responses as well as an important role in the transition between cognitive tasks and not just resting state (Smith, et al., 2018; Vatansever, et al., 2017). Much research is underway to better understand the role and function of the DMN.
Neuroscience research on social cognition and learning shows us how social interactions are important in learning, and how they seem to be required for learning language in infants (Kuhl, 2011). From this literature we may gain ideas about how to design cyberlearning systems in ways that will facilitate the socially-contingent aspects of learning. This, in turn, may help improve learning outcomes (Lieberman, 2013; Lieberman, 2012; Davachi, Mitchell, & Wagner, 2003; Mitchell, Macrae, & Banaji, 2004). Much work in the learning sciences is guided by the importance of social interactions (Lave & Wenger, 1991; Vygotsky, 1978) and research findings from neuroscience that show how “our brains are wired to connect” provide additional evidence for the importance of social interactions in learning and that learning is a very social endeavor for humans (Lieberman, 2013).
Much research has been done on the emotional response of stress, since it influences learning in profound ways. Early stressful life situations, such as poverty or trauma to the child or in the family, can cause delays to the development of executive functions (Barr, 2018). From research we learn that stress can be toxic and alter brain structures. Animal models suggest stress leads to excess corticosterone secretion and that leads to neurotoxicity in areas of the brain such as the hippocampus and prefrontal cortex, two areas involved in memory and executive function (Carrion & Wong, 2012). The way caring adults (parents, teachers, or others who are invested) respond can have positive or negative effects. The research on stress and emotion from the neuroscience perspective should be considered by learning researchers since there may be important implications for cyberlearning work.
Key Lessons
In this section, we discuss lessons learned from projects that have helped show the benefits of neuroscientists and learning or education researchers working together.
Computer Games, School Learning, and Neuroscience. Providing evidence of changes in the brain, McCandliss (2010) reports on results from a randomized control trial where kindergarten children played Graphogame, a game to help children master the association between a letter and its corresponding sound. Behind the scenes, algorithms analyzed a child’s performance to provide lessons that were challenging and engaging but not too difficult to be frustrating. Repeated measures fMRI showed that in the course of 8 weeks with on average 224 minutes of gameplay, the “brain circuit of the visual system and the language system” that is necessary for reading developed more in those who played the game (Brem et al., 2010). Reading researchers can also see when the reading circuit isn’t developed and new interventions can be developed. Research by Bers and colleagues is using fMRI to examine the cognitive and neural basis of computer programming in young children and how it engages the brain regions used in language learning (fronto-temporal) vs. general problem solving (fronto-parietal) to better understand effective learning trajectories.
Spatial Thinking Skills and STEM Achievement. Some evidence links spatial ability with future STEM attainment. Understanding the mechanism for why spatial skills help in future STEM attainment could lead to new interventions to help close the gap between those who achieve later success and those who don’t. For example, an NSF project led by Adam Green is examining the effects of spatial thinking skills on high school students studying geoscience and whether spatial training might reduce gender differences. In particular, the project is looking at neural, behavioral, and educational data from students in a geospatial course designed to improve spatial thinking with such data from peers in a non-spatially-based advanced STEM course. This project bridges classroom experiences with neuroscience by doing pre and post course MRIs (both functional and structural) and serves as one model for doing work that brings together the classroom and neuroscience.
Embodiment and What it Means to Have an Embodied Learning Experience. Recent neuroscience research has shown that thinking––even in domains considered very conceptual and symbolic (e.g., mathematics)––is linked to an embodied understanding through our sensory motor system (Gallese & Lakoff, 2005; Beilock, 2016). Similar to research showing that emotion and cognition are linked; for example, imaging studies show how sensory systems such as vision and touch integrate with each other and with brain systems typically considered to be “cognitive” (e.g., those involved in conceptual understanding, planning, and imagination; Gallese & Lakoff, 2005). NSF cyberlearning researchers Abrahamson and Lindgren (2014) discuss embodiment and embodied design in learning activities and environments; see also Lindgren’s GRASP project.
Virtual Reality and Neuroscience. Cyberlearning and neuroscience are both investigating virtual reality (VR). Concepts developed in cognitive neuroscience are important to understand and improve VR technology (Herbelin, Salomon, Serino, & Blanke, 2016). Some people call VR an embodied technology for the ability it has to give the user an experience of “presence” in a non-physical world and can allow for immersive experiences not possible in the real world. Since VR can manipulate perception and engagement, VR may lead to different ways of learning. Findings from neuroscience and learning are likely to inform one another and lead to a more convergent understanding of learner perception in VR.
Multimodal Research. Portable measures of brain activity such as fNIRS or EEG, and eye tracking, have led to studies of the mind-body connection through multimodal analysis as well as neurogaming research and development. Multimodal analysis uses data detected from different modalities (e.g., face, voice, posture, text) and physiological measures to create a more nuanced picture of the effects of different environmental stimuli and activities on the learner. By examining the time course of changes in activation across different streams of data from physiological sensors and data from logs of clicks that are generated in games or other digital environments, researchers can better understand the information processing occurring in the brain. The data may converge to relay a coherent image of a learner’s state, or indicate discrepancies that may not be apparent. Emerging neurocognitive measures are being developed for working memory capacity, the role of the frontal lobe in tasks, and cognitive load using EEG, pupillometry, eye tracking, and fNRIS (Meiri, et al., 2012; Klinger, 2010; Antonenko, Paas, Grabner, & Van Gog, 2010).
An especially relevant area for cyberlearning is multimodal analysis of user data in human computer interaction studies associated with the development of affective computing interfaces, which seek to use information about a user’s emotional state to better tailor a response. Predictions of emotional states can be made through various modality measures and multimodal analysis. With the increasing affordability and portability of EEG, researchers can now use patterns of brain activity as a modality measure in response to specific stimuli or events using event-related potentials (ERPs), which may lead to more accurate assessments of an individual’s emotional state. Theoretical understanding of the response of the brain during feedback-based learning is now mature enough to begin looking at these ERPs in real-world learning situations. Researchers are also using multimodal analysis to study collaborative learning and socio-emotional experiences through changes in gaze, gesture, and posture (Worsley, 2017). Multimodal research, generally, could help cyberlearning researchers better understand what is happening in learners during different individual and collaborative tasks and in different environments. A recent review by Lane & D’Mello (2019) discusses physiological monitoring and intelligent learning environments.
Issues
In 1997, the gap between education and neuroscience was said to be too wide to bridge (Bruer, 1997). In 2008, Varma and colleagues discussed scientific concerns around methods, data, theory, and philosophy as well as more pragmatic concerns about costs, timing, locus of control, and pay-offs as differences and opportunities. Twenty years after the discussion of the “too wide” of gap, there are still discussions about differences and very real scientific and pragmatic concerns, but the literature suggests that the gap is narrowing. There has been a real “initial attempt to locate educational neuroscience within the learning sciences” even if it is still very nascent (Bruer, 2016).
In a recent debate, Bowers (2016), Gabrieli (2016), and Howard-Jones and colleagues (2016) exchanged their perspectives on the promise of educational neuroscience to inform education. Bowers (2016) initially argued that neuroscience (cognitive neuroscience) would not be useful to improving teaching in the classroom or for the development of interventions for those with learning difficulties. He states that neuroscience does not add anything to the enterprise above what psychology already does, and goes further to claim that some things neuroscience tells us are “trivial” (e.g., the importance of sleep in the learning process and that a child who lives in fear will have a hard time learning). Gabrieli counters that educational neuroscience is better conceived as a basic science that seeks to inform education topics, such as the relationship between brain development and learning differences, rather than being focused on practical applications to improve classroom teaching more immediately. Gabrieli maintains that research in educational neuroscience should not be evaluated based on immediate practical results. On the other hand, understanding the relationship between cognitive systems and emotional systems during the learning process––and designing environments to support those processes––seems like important convergent work for neuroscience and other educational research.
A bridge between neuroscience and education is starting to occur, but still in early stages. Tommerdahl (2010) suggested a way for thinking about a bridge between neuroscience and education and proposed a model to link neuroscience, cognitive neuroscience, psychological theories, educational theory, and the classroom. She proposed that a translation has to be done through those five levels to have rigorous, high quality methods for the classroom that work well in the classroom. Schwartz, Blair, and Tsang (2012) discuss two ways for bridging between education and neuroscience. One way, the most prevalent way, is when the neuroscientist looks for potential applications of their work in education; the second way is when educational researchers take theoretical problems to neuroscience and work convergently with neuroscientists to see if they can solve these together. Like Tommerdahl, Schwartz and colleagues also suggest that the different levels, from neuroscience to the classroom, need to explain the same phenomenon and make links between the different levels. Both papers argue for the importance of this convergence.
“Neuromyths” that are perpetuated by popular culture add to common misunderstandings about the brain and learning. Commercial “brain-based” products promising improved knowledge and cognition (often in an easy, fun manner) with no evidence behind their claims take advantage of neuromyths. Some common examples of such myths include thinking that individuals only use 10% of their brain, individuals are “left-brained” or “right-brained”, or that listening to music by Mozart will increase intelligence. One approach to linking education and neuroscience has been to give teachers and practitioners a better understanding of neuroscience so they can determine what findings from neuroscience research are scientifically rigorous and may be most applicable to their classroom practice (Dubinsky, 2010).
If practitioners, parents, and administrators have a better understanding of how the brain works, they can help resist the “brain enhancing” products that have been inaccurately labeled as “backed by brain sciences” by marketing departments (Pasquinelli, 2012). Many of these products have not had rigorous testing and may or may not work. There are potential products that could help people learn or help them focus their executive function abilities. Ideally, research from neuroimaging could help create educational interventions for reading and mathematics (e.g., Boets et al, 2013; Hoeft, et al, 2011; Schlagger & McCandliss, 2007) but much of this research is still in development. However, there is a need to make sure that we make changes based on evidence and understand if they work in classrooms and what they bring to the learning process. In this, teachers and learning scientists should be an integral part of the process. Cyberlearning research opens the door for studying the promise and efficacy of applying new neuroscience technologies and methods in real world learning situations, including classrooms and informal settings.
Projects
Examples of NSF Cyberlearning projects that overlap with topics discussed in this primer.
Neuroscience
Multimodal Data/Environments
Other related projects:
- Collaborative Research: Revealing the Invisible: Data-Intensive Research Using Cognitive, Psychological, and Physiological Measures to Optimize STEM Learning uses neuro-imaging to measure student engagement and learning (NSF grants 1417967, 1417456, 1418122)
- GRASP (GestuRe Augmented Simulations for supporting exPlanations) is an NSF-funded collaboration between the University of Illinois at Urbana-Champaign and Concord Consortium that aims to understand the role that gestures play in reasoning about critical concepts in science.
- SL-CN: Contributions of Executive Function Subdomains to Math and Reading Cognition in the Classroom examines how executive functions contribute to academic performance and whether weak EFs can be enhanced with cognitive training.
- Cognitive and Neural Indicators of School-based Improvements in Spatial Problem Solving (NSF 1420481) and Neural and Cognitive Strengthening of Conceptual Knowledge and Reasoning in Classroom-based Spatial Education (NSF 1661065) examine the link between spatial ability and future STEM attainment and how spatial training may reduce gender differences.
Resources
CIRCL Webinar: Neuroscience and Cyberlearning: A Convergence Conversation
NSF
Journals
- Mind, Brain, and Education
- .Social, Cognitive, Affective Neuroscience (SCAN)
- Trends in Neuroscience and Education
Associations
- American Educational Research Association (AERA) Special Interest Group on Brain, Neuroscience and Education
- British Educational Research Association (BERA) Special Interest Group on Neuroscience & Education
- Cognitive Neuroscience Society (CNS)
- International Mind, Brain and Education Society (IMBES)
- Learning & the Brain Conference
Readings
Abrahamson, D., & Lindgren, R. (2014). Embodiment and embodied design. The Cambridge handbook of the learning sciences, 2, 358-376.
Anguera, J.A., Boccanfuso, J., Rintoul, J.L., Al-Hashimi, O., Faraji, F., Janowich, J., Kong, E., Larraburo, Y., Rolle, C., Johnston, E. and Gazzaley, A. (2013). Video game training enhances cognitive control in older adults. Nature, 501(7465), 97-101.
Antonenko, P., Paas, F., Grabner, R., & Van Gog, T. (2010). Using electroencephalography to measure cognitive load. Educational Psychology Review, 22(4), 425-438.
Barr, D. A. (2018). When trauma hinders learning. Phi Delta Kappan, 99(6), 39-44.
Beilock, S. (2015). How the body knows its mind: The surprising power of the physical environment to influence how you think and feel. Simon and Schuster.
Black, J. M., Myers, C. A., & Hoeft, F. (2015). The utility of neuroimaging studies for informing educational practice and policy in reading disorders. New directions for child and adolescent development, 2015(147), 49-56.
Brem, S., Bach, S., Kucian, K., Kujala, J. V., Guttorm, T. K., Martin, E., … & Richardson, U. (2010). Brain sensitivity to print emerges when children learn letter–speech sound correspondences. Proceedings of the National Academy of Sciences, 107(17), 7939-7944.
Boets, B., de Beeck, H. P. O., Vandermosten, M., Scott, S. K., Gillebert, C. R., Mantini, D., … & Ghesquière, P. (2013). Intact but less accessible phonetic representations in adults with dyslexia. Science, 342(6163), 1251-1254.
Bowers, J. S. (2016). The practical and principled problems with educational neuroscience. Psychological Review, 123(5), 600-612.
Bruer, J. T. (2016). Where Is Educational Neuroscience?. Educational Neuroscience, 1, 2377616115618036.
Bruer, J. T. (1997). Education and the brain: A bridge too far. Educational researcher, 26(8), 4-16.
Carrion, V. G., & Wong, S. S. (2012). Can traumatic stress alter the brain? Understanding the implications of early trauma on brain development and learning. Journal of adolescent health, 51(2), S23-S28.
Damasio, A. R. (1999). The feeling of what happens. New York, NY: Harcourt.
Davachi, L., Mitchell, J. P., & Wagner, A. D. (2003). Multiple routes to memory: distinct medial temporal lobe processes build item and source memories. Proceedings of the National Academy of Sciences, 100(4), 2157-2162.
Dubinsky, J. M. (2010). Neuroscience education for prekindergarten–12 teachers. Journal of Neuroscience, 30(24), 8057-8060.
Eden, G.F., Jones, K.M., Cappell, K., Gareau, L., Wood, F.B., Zeffiro, T.A., Dietz, N.A., Agnew, J.A., & Flowers, D.L. (2004). Neural changes following remediation in adult developmental dyslexia. Neuron, 44(3), 411-422.
Gabrieli, J. D. (2009). Dyslexia: a new synergy between education and cognitive neuroscience. science, 325(5938), 280-283.
Gabrieli, J. D. (2016). The promise of educational neuroscience: Comment on Bowers (2016). Psychological Review, 123(5), 613-619.
Hofmann, W., Schmeichel, B. J., & Baddeley, A. D. (2012). Executive functions and self-regulation. Trends in cognitive sciences, 16(3), 174-180.
Gallese, V., & Lakoff, G. (2005). The brain’s concepts: The role of the sensory-motor system in conceptual knowledge. Cognitive neuropsychology, 22(3-4), 455-479.
Goswami, U (2004). Neuroscience and education. British Journal of Educational Psychology, 74 (1): 1–14. doi:10.1348/000709904322848798. PMID 15096296.
Herbelin, B., Salomon, R., Serino, A., & Blanke, O. (2016). 5 Neural Mechanisms of Bodily Self-Consciousness and the Experience of Presence in Virtual Reality. Human Computer Confluence Transforming Human Experience Through Symbiotic Technologies, 80.
Hoeft, F., McCandliss, B. D., Black, J. M., Gantman, A., Zakerani, N., Hulme, C., … & Gabrieli, J. D. (2011). Neural systems predicting long-term outcome in dyslexia. Proceedings of the National Academy of Sciences, 108(1), 361-366.
Howard-Jones, P. A. (2014). Neuroscience and education: myths and messages. Nature Reviews Neuroscience, 15(12), 817-824.
Howard-Jones, P. A., Jay, T., Mason, A., & Jones, H. (2015). Gamification of learning deactivates the default mode network. Frontiers in psychology, 6.
Howard-Jones, P. A., Varma, S., Ansari, D., Butterworth, B., De Smedt, B., Goswami, U., … & Thomas, M. S. (2016). The principles and practices of educational neuroscience: Comment on Bowers (2016). Psychological Review, 123(5), 620-627.
Lane, H. C., & D’Mello, S. K. (2019). Uses of Physiological Monitoring in Intelligent Learning Environments: A Review of Research, Evidence, and Technologies. In Mind, Brain and Technology (pp. 67-86). Springer, Cham.
Lieberman, M. D. (2012). Education and the social brain. Trends in Neuroscience and Education, 1(1), 3-9.
Lieberman, M. D. (2013). Social: Why our brains are wired to connect. OUP Oxford.
Lindquist, K. A., Wager, T. D., Kober, H., Bliss-Moreau, E., & Barrett, L. F. (2012). The brain basis of emotion: a meta-analytic review. Behavioral and brain sciences, 35(3), 121-143.
Lindquist, K.A., & Barrett, L. F. (2008). Constructing emotion: The experience of fear as a conceptual act. Psychological Science, 19, 898–903.
Klingner, J. (2010). Measuring cognitive load during visual tasks by combining pupillometry and eye tracking (Doctoral dissertation, Stanford University).
Kuhl, P. K. (2011). Early language learning and literacy: neuroscience implications for education. Mind, Brain, and Education, 5(3), 128-142.
Mangels, J. A., Good, C., Whiteman, R. C., Maniscalco, B., & Dweck, C. S. (2012). Emotion blocks the path to learning under stereotype threat. Social Cognitive and Affective Neuroscience, 7(2), 230-241.
Mars, R.B., Neubert, F.X., Noonan, M.P., Sallet, J., Toni, I. and Rushworth, M.F., 2012. On the relationship between the “default mode network” and the “social brain”. Frontiers in human neuroscience, 6, p.189.
McCandliss, B. D. (2010). Educational neuroscience: The early years. Proceedings of the National Academy of Sciences, 107(18), 8049-8050.
Meiri, H., Sela, I., Nesher, P., Izzetoglu, M., Izzetoglu, K., Onaral, B., & Breznitz, Z. (2012). Frontal lobe role in simple arithmetic calculations: An fNIR study. Neuroscience letters, 510(1), 43-47.
Mitchell, J. P., Macrae, C. N., & Banaji, M. R. (2004). Encoding-specific effects of social cognition on the neural correlates of subsequent memory. Journal of Neuroscience, 24(21), 4912-4917.
Moisala, M., Salmela, V., Hietajärvi, L., Salo, E., Carlson, S., Salonen, O., … & Alho, K. (2016). Media multitasking is associated with distractibility and increased prefrontal activity in adolescents and young adults. NeuroImage, 134, 113-121.
Pasquinelli, E. (2012). Neuromyths: why do they exist and persist? Mind, Brain, and Education, 6(2), 89-96.
Pessoa, L. (2008). On the relationship between emotion and cognition. Nature reviews neuroscience, 9(2), 148-158.
Raichle, M. E. (2015). The brain’s default mode network. Annual review of neuroscience, 38, 433-447.
Rizzolatti, G., Fogassi, L., & Gallese, V. (2006). Mirrors in the mind. Scientific American, 295(5), 54-61.
Rydell, R. J., McConnell, A. R., & Beilock, S. L. (2009). Multiple social identities and stereotype threat: Imbalance, accessibility, and working memory. Journal of personality and social psychology, 96(5), 949-966.
Schlaggar, B. L., & McCandliss, B. D. (2007). Development of neural systems for reading. Annual Review of Neuroscience, 30, 475-503.
Schwartz, D. L., Blair, K. P., & Tsang, J. M. (2012). How to build educational neuroscience: Two approaches with concrete instances. British Journal of Educational Psychology Monograph Series II, (8) 9-27.
Shaywitz, S. E., Morris, R., & Shaywitz, B. A. (2008). The education of dyslexic children from childhood to young adulthood. Annual Review of Psychology, 59, 451-475.
Singer, T., Seymour, B., O’Doherty, J. P., Stephan, K. E., Dolan, R. J., & Frith, C. D. (2006). Empathic neural responses are modulated by the perceived fairness of others. Nature, 439(7075), 466-469.
Slovic, P., Finucane, M. L., Peters, E., & MacGregor, D. G. (2007). The affect heuristic. European journal of operational research, 177(3), 1333-1352.
Szűcs, D., & Goswami, U. (2007). Educational neuroscience: Defining a new discipline for the study of mental representations. Mind, Brain, and Education, 1(3), 114-127.
Tardif, E., Doudin, P. A., & Meylan, N. (2015). Neuromyths among teachers and student teachers. Mind, Brain, and Education, 9(1), 50-59.
Tommerdahl, J. (2010). A model for bridging the gap between neuroscience and education. Oxford Review of Education, 36(1), 97-109.
Varma, S., McCandliss, B. D., & Schwartz, D. L. (2008). Scientific and pragmatic challenges for bridging education and neuroscience. Educational researcher, 37(3), 140-152.
Vatansever, D., Menon, D. K., & Stamatakis, E. A. (2017). Default mode contributions to automated information processing. Proceedings of the National Academy of Sciences, 114(48), 12821-12826.
Worsley, M. (2017). Multimodal analysis. In J. Roschelle, W. Martin, J. Ahn, & P. Schank (Eds.), Cyberlearning Community Report: The State of Cyberlearning and the Future of Learning With Technology (pp. 46-50). Menlo Park CA: SRI International.
Weissman, D. G., Conger, R. D., Robins, R. W., Hastings, P. D., & Guyer, A. E. (2018). Income change alters default mode network connectivity for adolescents in poverty. Developmental Cognitive Neuroscience, 30, 93-99.
Yeager, D., Henderson, M., Paunesku, D., Walton, G., D’Mello, S., Spitzer, B., Duckworth, A. (2014) Boring but Important: A Self-Transcendent Purpose for Learning Fosters Academic Self-Regulation, Journal of Personality & Social Psychology, 107(4), 559-580.
Citation
Primers are developed by small teams of volunteers and licensed under a Creative Commons Attribution 4.0 International License. After citing this primer in your text, consider adding: “Used under a Creative Commons Attribution 4.0 International License (http://creativecommons.org/licenses/by/4.0/).”
Suggested citation:
Fusco, J., Asbell-Clarke, J., & Sibuma, B. (2019). CIRCL Primer: Neuroscience and Cyberlearning. In CIRCL Primer Series. Retrieved from http://circlcenter.org/neuroscience-and-cyberlearning
Special thanks to Ibrahim Dahlstrom-Hakki, Britte Cheng, Lorna Quandt, and Patti Schank for reviewing and suggesting edits to this primer, and to Melina Uncapher for discussions around translating neuroscience and learning sciences research into practice.