Authors: Jeremy Roschelle & Patricia Schank; Contributors: Judi Fusco & Wendy Martin
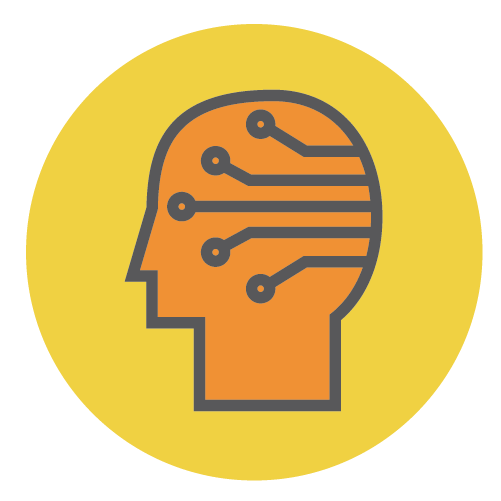
Definition
Cyberlearning projects advance state of the art technologies and techniques from computer science, data science, robotics, and other areas. In conjunction with learning scientists and experts in equity and in particular subject matter or educational contexts, cyberlearning projects seek to advance our understanding of these technologies and techniques in the context of human learning challenges. In reviewing the portfolio, the most common types of technologies and techniques are:
- Intelligent Tutoring Systems, in which a computational agent supports and guides students as they work to learn challenging subject matter.
- Machine Learning, where statistical techniques are applied to infer patterns in large data sets about learner behavior and outcomes. Insights and techniques for guiding learner’s behavior to desired outcomes are sought.
- Speech, Vision, and Natural Interaction, where machine learning and other techniques are specifically applied to analyze human speech, visual scenes in which learners participate, eye-gaze, etc. and (in cases) to synthesize naturalistic interactions with learners in the same modalities.
- Social Robotics and Avatars, where above techniques are applied to provide an artificial learning partner to a student or group of students.
The Artificial Intelligence & Education (AI&Ed) community is long-standing and accomplished, with its own society, journals, and multiple funding streams. As participants in the cyberlearning community, experts in the above topics were somewhat less present in the first 3-4 years, but participation increased strongly thereafter. Rather than simply advancing Intelligent Tutoring Systems (ITS) technology with AI, cyberlearning projects combine AI with learning theories to create “ambitious mashups.” Examples include exploring hybrid systems in which people and AI agents work together, focusing on social learning (classic AI & Ed research was somewhat more individual-oriented). Cyberlearning AI projects also support teachers and expand to applications like social robots for learning Chinese, AI supports for workforce learning, and an invention coach (whereas early ITS applications were often oriented to more bounded problem solving in math and science). Thus, the portfolio of cyberlearning projects push beyond AI beyond the boundaries of a conventional ITS.
Please see the full report for additional information on how this theme changed over time in cyberlearning research and for some questions that arose as we investigated the theme.
Project Examples and Resources
Intelligent Tutors
- 36 Projects
- 16 sessions at cyberlearning convenings
Stimulating Quotes and Snippets
- “The time for real time feedback is in the moment, while the student is learning. It doesn’t make sense to allow students to go through all the inquiry phases and then a week or two from then get back to them. It’s so important that students get the feedback in real-time and the teacher can stop the class in real-time. It affects a teacher’s instruction in a way that supports inquiry learning as it needs to be supported, in the moment. It’s like apprenticeship learning where you provide situated experiences with feedback in real time, with the apprentice working side by side with the mentor; that’s the big benefit we see from Inq-ITS & Inq-Blotter.” – Janice Gobert
- “Intelligent tutoring systems are efficient for some procedural skills, freeing teachers to spend time on other needs or with other students to provide more individualized support––or giving them time to plan the next lesson.” – Claudia Mazziotti
Example Project Abstracts:
- EXP: Modeling Perceptual Fluency with Visual Representations in an Intelligent Tutoring System for Undergraduate Chemistry
- EXP: Helping Teachers Help Their Students: Teachers’ Use of Intelligent Tutoring Software Analytics to Improve Student Learning.
- EXP: Students Authoring Intelligent Tutoring Systems for Constructing Models of Ill-Defined Dynamic Systems
Related Primers/Spotlights/Reports:
Spotlight: The Invention Coach
Showcase Videos and/or Gallery Posters:
- Inq-ITS: Using Data Mining to Assess Science Skils/Practices
- ASSISTments and Sharing of work by Teachers
Cross Connections:
- Assessment
- Learning Theories: Cognitive
Machine Learning
- 17 Projects
- 4 sessions at cyberlearning convenings
Stimulating Quotes and Snippets
- (mashup) “We’re trying to take all of these [multimodal data streams] together and then machine learn models of engagement in order to examine how we can empirically understand what it means for museum visitors to be engaged. The deliverable at the end of the project is a deeper understanding of learning.“ – James Lester
- “Machine learning and its applications to computer vision, speech analysis, and natural language processing offers powerful ways to model and optimize human learning. These new opportunities will continue to grow as sensors (cameras, microphones, wearable devices) proliferate. However, due to the complexity of human learners and school classrooms, there are still many important computational challenges to be solved. Moreover, it is important to consider how computational analyses can be conducted while respecting people’s privacy.” – Jacob Whitehill & Anand Ramakrishnan
Example Project Abstracts:
- EAGER: Collaborative Research: Automated Instruction Assistant for Argumentative Essays
- Collaborative Research: Human-Technology Partnership Supporting Career Path Exploration and Navigation</li>
Related Primers/Spotlights/Reports:
Showcase Videos and/or Gallery Posters:
- Multimodal Machine Learning for Automated Classroom Feedback
- Using Machine Learning to Put Teachers in the DRIVER-SEAT
- The Control-Value Theory of Achievement Emotions and Attritions in MOOCs: Machine Learning Perspectives
Cross Connections:
- Methods (in general)
- Multimodal analytics
Speech, Vision, Naturalistic Interaction
Stimulating Quotes and Snippets
- “Educational research has barely begun exploring the potential of speech technology for areas as diverse as collaboration, argumentation, discourse analysis, emotion, and engagement, not to mention discourse and conversation among students, teachers, and mentors that forms the core of teaching and learning.” – Cynthia D’Angelo
- “’I’d love to see a virtual human or a robot be instrumented and connected to an instrumented data scientist and get to a point where they are able to meta-reason — that is, turn to the data scientist and say something like, “Listen, there is a lot of physiological data that was observed from this human learner, but you’re not paying attention to it. Why not?” Can we have a productive collaboration between virtual and human data scientists?” – Roger Azevedo
Example Project Abstracts:
- CAREER: Dialogue Engagement for Educational Robots
- EAGER: Collaborative Research: Interactive Dialog Agents for Social Language Development and Listening Comprehension
- EXP: Partners in Learning: Building Rapport with a Virtual Peer Tutor
Related Primers/Spotlights/Reports:
- Primer: Speech Technologies & Learning
- Spotlight: Scaffolding Learners’ Self-Regulation Skills to Optimize Science Learning
- Spotlight: Interactive Robot for Learning Chinese
- Workshop: Robots, Young Children, & Alternative Input Methods
Showcase Videos and/or Gallery Posters:
- Inclusive Design for All Learners (IDEAL)
- Predicting student collaboration from speech
- Teaching Cybersecurity with Networked Robots
Cross Connections:
- Collaborative Learning
- Social-emotional learning
Potential Platforms
- ASSISTments
- LearnLab
- EdX and other MOOCs
- Algebra Nation and other curricula
- Connected Worlds
- Future Worlds
Exit Survey Highlights (30 total responses)
- Projects with staff including AI/Machine learning researchers: 13
- Projects tagged as AI/ML: 6
- Given that 13 projects include AI researchers, but only 6 chose to tag their projects as AI/Machine Learning, it appears that these researchers are working on projects that focus on themes outside of just AI/Machine Learning.
- Gender of PIs: 3/6 Female
CL is a place where women are well-represented among PIs - Project Implementation Setting:
- 5/6: Formal school setting
- 1/6: Formal and Informal learning setting; museum/science center
- 1/6: Workplace
- Special populations targeted:
AI/Machine learning projects did not specifically target any special populations - Explicit focus on cyberlearning in preparation for and within the context of the work setting: 4/6
- 3/4 specifically related to supporting the current and future work of teachers in classrooms and other related settings; 1/4: Design and develop future learning environments to educate/re-educate workers for new worker environments and experiences in collaboration with advanced technology.
- Project included teacher/practitioner partnerships: 5/6
- Project included 2 or more grad students on project staff: 5/6
- PI proposals and awards
- PI received new Cyberlearning award: 4
- PI submitted proposal to Future of Work program: 1
- PI received an NSF award for a program other than Cyberlearning: 3
- Project Publications:
- 2/6 projects indicated that they published project findings in scholarly journals, including: Mind Brain and Education; Journal of Computer Assisted Learning; Multimodal Technologies and Interaction