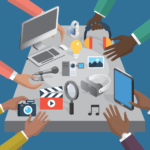
Overview
This report reflects on progress from over eight years of research projects in the cyberlearning community. The community involved computer scientists and learning scientists who received NSF awards to investigate the design of more equitable learning experiences with emerging technology—focusing on developing the learning theories and technologies that are likely to become important within 5-10 years. In early 2020, our center’s team analyzed the portfolio of past and current projects in this community, and convened a panel of experts to reflect on important trends and issues. This site briefly presents the insights from this process; a more comprehensive account is available in the downloadable report.
What are Ambitious Mashups?
The concept of “ambitious mashups” describes what makes this collection of projects special and worthy of review. We first tried to categorize or tag the projects, but soon realized that many projects defied simple characterization. Projects did not fall into one major technology that they were exploring. Nor did they only refine one research method. Nor did they develop only one type of learning theory. The interdisciplinarity of the projects created new combinations of methods to address problems. Equity concerns that arose were also not singular.
The projects we reviewed were mashups—they combined many elements in a novel integration, to explore a promising approach to a particular learning goal or context. A project, for example, might involve elements of artificial intelligence, virtual reality, and dynamic simulations. It might involve theories of collaborative learning, learning from representations and embodied learning. Methods could involve analyzing discourse, embodied cognition, and learning analytics.
The projects also were very ambitious. These were not integrations of the most established aspects of each technology, theory, or method. Rather they were pushing for frontiers—trying to explore combinations which do not easily co-occur with today’s learning environments, but might co-occur in the future. Projects were also ambitious in terms of wanting to study big learning goals; they did not focus on incremental improvements in test scores. Rather they were working to open up difficult subjects so that more students could learn them and/or challenging who can learn important scientific topics in ways that feel personally relevant and connected.
Cyberlearning projects design ambitious mashups and they investigate what happens when learners inhabit them. The output isn’t usually intended to be a “solution” that “scales up”—that would be a near-term goal to apply technology to learning. Rather the output is usually intended to reach a better understanding of a technology, a theory, a method, or an approach to equity—an understanding that might be applied more broadly in the future as it arrives. Indeed, cyberlearning projects also have a strong focus on groups that are underrepresented in STEM professions, with projects often stating a specific focus on underrepresented minorities, women/girls, low-performing schools, low-income settings, students with a disability, or students who are learning English.
Theme Identification & Analysis Methodology
Our team chose and analyzed key themes for the Reflections Briefs and advisory meeting using a hybrid approach of bottom-up (data driven) and top-down (knowledge driven) methods.
The bottom-up approach leveraged tags derived from the cyberlearning portfolio. All NSF-funded Cyberlearning projects on the CIRCL website are tagged by one or more of roughly 50 tags. These tags were generated by the CIRCL portfolio analysis team based on content reviews of CFLT projects across the years. Proposed tags are also included in project intake and exit surveys sent to PIs, who are asked to indicate which tags apply to their projects and/or suggest new tags. Since not all PIs respond to these surveys, the CIRCL team reviewed project abstracts and assigned tags when they were needed. Further, in 2018, the team conducted a major review of the full tag set and did some consolidation and retagging.
For the Reflections analysis, the team counted unique tags across projects, created clusters of related tags and projects, discussed the counts and clustering results, and selected a final set of themes for analysis:
- Artificial Intelligence & Education
- Learning Theories
- Research Methods
- Out-of-school-time (OST) Learning
- Trends at NSF and Beyond
The first four themes emerged primarily from (bottom up, data driven) coding frequencies, but the CIRCL team’s (top down) knowledge and experience doing the Cyberlearning Community Report also helped us think through important themes and meaningful clusters, influencing the process. The fifth theme, Trends at NSF and Beyond, was derived from our (knowledge-driven) discussions of the themes and our understanding of external trends such as NSF Big Ideas, neuroscience and learning, and issues around equity and access.
To continue reading the report, click on topics on the left side of this page.