CIRCL Spotlights illuminate a diverse set of projects funded by NSF. Want us to spotlight your project? Contact CIRCL to contribute your story.
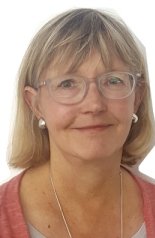
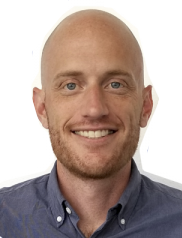
This spotlight is based on a blog post and interview with Andy Krumm, Director of Learning Analytics Research at Digital Promise, and Barbara Means, Executive Director of Learning Sciences Research at Digital Promise.
Portions reprinted from Learning Analytics Goes to School Blog Post with permission.
What is the big idea of your work?
Collaborations among researchers and practitioners have long been framed as an ideal, but the elements of what makes for an effective collaboration are often not well understood, and the funding for such work has been scarce. Recently, however, both government and private research funders have become interested in collaborations under the label of research-practice partnerships. As partnerships have proliferated, collective knowledge and wisdom about how to implement successful partnerships has grown.
Over our years of work with educational organizations, we’ve identified several factors that contribute to successful partnerships — such as the importance of forming trusting relationships between researchers and practitioners, using an explicit improvement method to guide research activities, and intentionally designing learning opportunities that build the capacity of both researchers and practitioners.
Our new book, Learning Analytics Goes to School, co-authored with Marie Bienkowski at SRI Education, describes a particular kind of partnership that is focused on leveraging complex data from various technologies to improve teaching and learning.
How can researchers, practitioners, and technology developers best use the wealth of data generated by the increasing use of technology in schools?
Related DOE OET Project
Title: Enhancing Teaching and Learning Through Educational Data Mining and Learning Analytics (Issue Brief)
Investigator: Marie Bienkowski, Mingyu Feng, Barbara Means
We’ve been among the many people wrestling with this question over the last five years, and our efforts have led us to a surprisingly simple answer: The best way to use data from digital learning environments for the purposes of educational improvement is in collaboration with the practitioners who will take action based on the data.
The book distills insights gathered over the course of dozens of cycles of inquiry involving work with K-12 schools, community colleges, and youth-serving organizations, and offers an account of the supporting conditions and key phases involved in what we refer to as collaborative data-intensive improvement.
Tell us more about your book?
Related NSF Projects
Title: EAGER: Elaborating Data Intensive Research Methods through Researcher-Practitioner Partnerships (NSF Award Details)
Investigator: Andrew Krumm, Mingyu Feng, Alex Bowers
Title: A Data-Intensive Exploration of the Links between SES and STEM Learning (NSF Award Details)
Investigator: Andrew Krumm
We begin the book with an introduction to the developing fields of learning analytics and educational data mining and end it with calls to action for new as well as experienced researchers, practitioners, and technology developers. In between, we provide accessible descriptions of the new sources of data fueling data-intensive research in education, the application of new analytical techniques, and the importance of students’ privacy in using data for research and improvement. We’ve worked to avoid pat exhortations to be “data driven” and instead to provide a historical account of data use in schools to ground our approach for working directly with practitioners to analyze, make sense of, and take action on data spread across various learning technologies and databases.
Along with useful background information and historical framings, we offer advice for launching data-intensive research-practice partnerships. Based on first-hand experiences leading multiple partnerships, we describe the importance of cultivating trust between researchers and practitioners, using explicit improvement methods, creating learning events for partnership members, and developing common workflows for sharing and analyzing data.
The core lesson is that data is a necessary but not sufficient condition for creating more equitable and effective learning environments. We hope researchers and practitioners alike can use the book as an on-ramp to the exciting possibilities of data-intensive research in education.
Where do you see your work going in the future?
Data-driven improvement requires more than just data and partnerships. In order to effectively work with data, researchers and practitioners need a technical infrastructure that supports secure partnership-driven, data-intensive research.
Many of the most powerful data analysis approaches require a workflow and technical infrastructure that many schools do not yet have. In general, this necessary infrastructure includes data analysis tools and storage that allow researchers and practitioners to jointly explore and model data originating from multiple sources. Currently, this work is either not occurring (i.e., data from multiple systems are not being merged and analyzed with state-of-the art statistical and machine learning approaches) or is carried out in labor intensive ways. Key to engaging in partnership-driven, data-intensive research involves reducing the movement of “big” datasets, supporting collaborative and distributed data analyses, and expediting the process by which practitioners access the products of analyses. We’re currently designing and pilot testing a cloud-based infrastructure that we can “spin up” for new partners or projects to offer partnerships a secure set of tools to engage in collaborative data-intensive research.
Resources
Krumm, A., Means, B., & Bienkowski, M. (2018). Learning Analytics Goes to School: A Collaborative Approach to Improving Education. Routledge.