Authors: Cynthia D’Angelo and Chad Dorsey
Printer-Friendly PDF | Google Doc for Comments | Questions? Contact CIRCL.
Overview
The classroom learning environment is filled with speech in all forms—classroom discourse has notably been called “the language of learning.” However, learning mediated by speech remains complex and daunting to investigate at any meaningful scale. Characterizing and evaluating processes from collaboration to argumentation to engagement currently demands analysis of mountains of audio and video data.
Today, speech recognition and analysis has come into its own as a highly advanced, data-intensive technological and engineering field, and some fruits of this work have become publicly visible via impressive tools such as Siri, Amazon Echo, and others. However, advances in speech technology remain underutilized by the education research community.
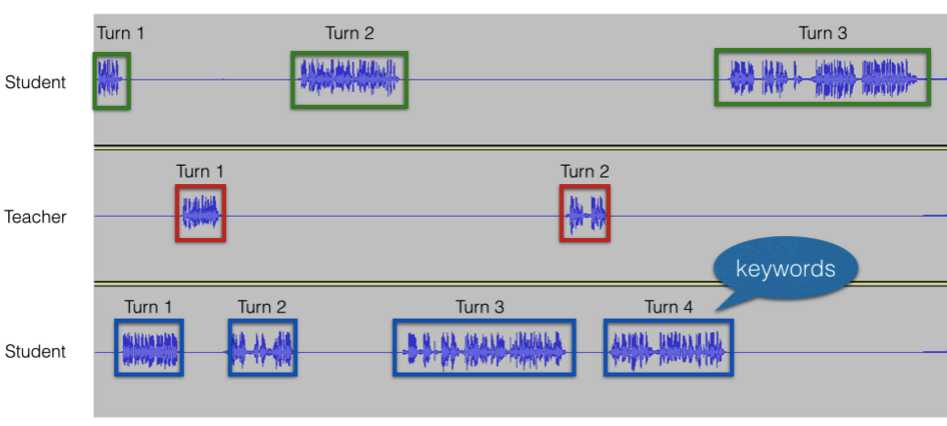
Speech activity detection (SAD) and diarization can be used to detect turn-taking, keywords, questions, total words spoken, and overlapping speech. See Webinar: Exploring the Promise of Speech Technology for Education Research.
Speech and language technologies (SLT) have reached the point where they offer untapped potential across a broad range of natural teaching and learning settings. These advanced applications, fueled mostly by DARPA projects and other national efforts, have made incredible strides toward recognition and classification of a wide set of features of spoken language. Current techniques to capture and characterize human speech and dialogue are well developed and in active use within many fields and applications. Advanced microphone technologies make extended audio capture effective and reliable, established noise-reduction and processing algorithms aid in automatic identification of speakers, and reliable algorithms enable automatic detection of everything from spoken questions and answers to emotion, sentiment, and use of specific content keywords.
Combining these capabilities with education research goals could unlock many possibilities. Targeted convergence research—consisting of the application of speech technologies for the capture, analysis, processing, and reporting on educational activities—could eventually encompass significant, wide-ranging applications. With support from and NSF-funded capacity-building grant, Chad Dorsey and Cynthia D’Angelo led focus group meetings with 24 education and speech researchers that resulted in the following visions for speech-enabled educational activities:
- A “Fitbit for teaching” that would provide teachers with an overview of their dialogic activity within different classes, including quantifications of conversational turns, numbers of questions asked or initiated by students, and classifications of the teacher’s response and verbal guidance types.
- A process for establishing speech-based learning analytics for collaboration, using the speech of small groups of students to determine the quality of each group’s collaboration and report to both teachers and researchers about collaboration quality and group dynamics.
- A process for automatically capturing and analyzing student discourse for argumentation indicators, including software that can identify and auto-extract a concentrated “highlight reel” of argumentation-rich instances across multiple small-group discussions.
- A means of performing longitudinal analysis of students and classrooms at scale, capturing weeks’ worth of voice data and processing it to look for variables otherwise out of reach because of human analysis constraints, such as how the use of domain-specific keywords evolve over time throughout a unit of instruction or the presence and implication of long-term variables such as instructional styles and average emotional character and sentiment of individuals and classrooms.
These goals are lofty, and many of them are years or even decades away. They will remain even farther out of reach, however, if the right imaginations and community-building activities are not properly engaged across the fields of speech engineering and educational research and the right data repositories and tools are not constructed.
A handful of projects and research groups are working on merging SLT and education research. These interdisciplinary partnerships have begun tapping into the potential of this genre of work, but there is much left to do.
Key Lessons
Current capabilities of speech analysis technology
Verbalized speech among multiple people contains a wealth of information that stretches far beyond the mere words uttered. Features such as prosody (tonality), rate of speech, loudness contours, and intonation patterns (e.g., questioning vs. statement), as well as more complex utterance styles such as sarcasm, reflect more than just propositional meaning. Patterns of interaction between or among speakers—such as who speaks more, latencies between conversational turns, disfluencies and discourse markers, or how often individual speakers overlap with each other or negotiate who gets the floor—provide rich information about individual speakers and about groups as a whole (e.g., Ford & Couper-Kuhlen, 2004; Sacks, Schegloff, & Jefferson, 1974).
Word and turn counts can paint a surprisingly detailed picture of interaction situations that stands to illuminate important aspects in educational research. Word and turn count analyses can be remarkably accurate and reliable metrics of basic dialogic interaction (Ziaei, Sangwan, & Hansen, 2014), and can be used to successfully distinguish leader-follower relationships in conversational exchange (Laskowski & Shriberg, 2012).
Question detection attempts to identify the location of questions in captured audio discourse. Lexical, syntactic, prosodic, and turn-taking features have been shown to be useful for accurately detecting questions (Kaushik, Sangwan, & Hansen, 2015a; Kolar, Lui, & Shriberg, 2010).
Algorithms can detect social engagement signals such as laughter and speakers’ sentiment — positive, negative, or neutral opinion in spoken data, as well as agreement or disagreement—increasingly reliably (Gupta, Audhkhasi, Lee, & Narayanan, 2013; L Kaushik, Sangwan, & Hansen, 2015b). Emotional “hot spots” in audio data can also be reliably determined and related to excitement, engagement, or level of social interactivity (Bou-Ghazale & Hansen, 2000; Hasan, Boril, Sangwan, & Hansen, 2013; Laskowski & Shriberg, 2012; Shokouhi, Sathyanarayana, Sadjadi, & Hansen, 2013; Wrede & Shriberg, 2003; Zhou, Hansen, & Kaiser, 2001).
The most publicly well-recognized realm of speech analysis today involves identification of spoken words themselves. Despite the success of consumer systems, full recognition of spoken language in naturalistic settings is still marked by high error rates. However, properly augmented keyword spotting systems can meaningfully identify specific sets of words (Akbacak, Burget, Wang, & Van Hout, 2013; Mandal et al., 2013; Sangwan & Hansen, 2010), even in extremely challenging conditions.
For more information on the state of speech technologies that are relevant to educational research, please refer to the CIRCL Webinar: Exploring the Promise of Speech Technology for Education Research.
Importance of partnerships and convergent science
Due to the integrated and convergent nature of this type of work, speech technology and learning research cannot be undertaken alone. Education researchers have expertise and understanding of the specific contexts in which this type of work will take place, as well as knowledge about which problems are more important to solve in this space. Speech researchers have expertise about the uses and limits of the technology at play, including how to collect the data and process it appropriately. In areas of innovation such as this, an integrated approach that can combine the expertises of both groups is needed in order to make progress.
As with any relationship, a good understanding of the values, languages, and commitments of the other side is needed, and compromises must be made to solve the common goal. For instance, when working in classrooms with children, there may be best practices of speech data collection that will not work easily with how teachers or children interact during a typical class. Education researchers must be able to communicate, for example, why it’s important to allow children to move around the room or why they don’t want to prevent children from talking to each other. On the other hand, speech researchers must be clear about why they need, for example, clean audio signals from individuals rather than groups of students. Finding a solution that will work for both sides is crucial, as is understanding the limits and caveats (or more difficult analysis work) that these kinds of solutions entail. The earlier in a partnership that communication around these important values and languages can be had, the better.
Low-hanging fruit for speech integration in ed research
Some of the settings and applications in educational settings that are most ready for the integration of speech technologies are: reading fluency, literacy and language acquisition, interaction with robots and other technological systems, collaboration, tutoring systems, assistive technologies, and working with early learners (using speech as an alternative input method).
Given all of the capabilities of speech technologies, a wide range of educational research areas are primed to advance with the application of spoken language technologies. We outline a few of these below.
Collaboration. Research has shown that face-to-face collaborative and cooperative learning is beneficial for students’ learning (Bricker, Tanimoto, & Hunt, 1998; Hymel, Zinck, & Ditner, 1993; Slavin, 1991). But assessing collaboration presents a quandary. Speech technology could offer a more quantified approach to assessing and/or understanding collaboration and its related properties of behaviors such as their relative frequency or prevalence, the sequencing of groups of these behaviors, and the timing of the behaviors within a given session of collaborative learning.
Argumentation and reasoning. Some of the more researched science-related practices in education are argumentation and reasoning. These are typically evaluated through writing, but helping students understand how to engage in these practices through dialogue and discussion is increasing important and lends itself to analyses of student (and teacher) speech. While qualitative methods such as the Claim-Evidence-Reasoning framework (McNeill, Lizotte, Krajcik, & Marx, 2006) reign supreme, argumentation and reasoning still present opportunities for the application of more automated techniques. Erduran, Simon, and Osborne (2004) demonstrate that rebuttals can be distinguished via keywords or phrases such as “but,” “I disagree with you,” or “I don’t think so,” and Mercer and Littleton (2004; 2007) and Herrlitz-Biro, Elbers and deHaan (2013) go further, identifying targetable indicator words (“because,” “I think,” “would,” “could,” “if,” “so,” etc.) explicitly connected with student reasoning and exploratory talk, quantifying length of student utterances in discussions, and showing that keywords in context can stand as useful markers of exploratory talk. Chi (2009) also classifies conceptually productive types of interactive student talk via patterns of utterance length and leader/follower exchange, both potentially automatable metrics.
Teacher questioning, facilitation, and classroom ecology. Teacher questioning and whole-class discussion is well studied, and many options exist for ready quantification. Simple counts of numbers of questions or relative time spent in question-asking are a longstanding, illuminative gauge of the tenor of pedagogy (Barnes, 1983; Levin & Long, 1981; West & Pearson, 1994). Wait time following questions has also proven to be both simple and powerful (Tobin, 1987; van Zee, Iwasyk, Kurose, Simpson, & Wild, 2001). Teacher-student questioning patterns, especially the initiate-respond-evaluate pattern (Cazden & Beck, 2003) are established and measurable. English language education research offers another rich source of techniques. Boyd and Rubin (2006) draw from student engagement and dialogue work to describe the importance of “student critical turns,” structurally coherent, socially engaged student utterances lasting more than 10 uninterrupted seconds. All lend themselves well to automated metrics and analysis.
Student motivation and engagement. Measurement of motivation and engagement is still in its early days, and much measurement relies upon student self-reports. A survey of 21 instruments for the measurement of student engagement found only four that used classroom observational models rather than student or teacher report, and identified them as limited and time-intensive (Fredricks et al., 2011). Clearly there is room for new metrics and quantification, and indeed Forbes-Riley and Litman (2004; 2005) report initial results indicating that the use of automated acoustic-prosodic profiles offer promising accuracy for supplanting manually labeled emotions.
Issues
Data sets and speech models
Speech research depends on having accurate and appropriate models of speech to power the analyses. These models are based on data sets (some shared across researchers) that have been collected over time in specific situations. For instance, there are data sets of adults during meetings (sitting around a table having a discussion) and data sets of people reading certain texts. All of these data sets inform what researchers know about how people say words and express emotion and intent through their voice.
Unfortunately, there are very few data sets of children, and the ones that do exist are limited in applicability for many of the contexts and problems of learning environments. Because of this dearth of data sets, the models of children’s speech are also very limited. Children’s voices are quite different than adults in many ways. First, their voices are changing over time, and in some cases over short periods of time. Second, younger children’s voices sound similar to one another, making it difficult for speaker recognition technology. All of these issues are compounded by the other issues that also apply to adult speech: there is significant linguistic variation across regions of the world and a substantial amount of data must be collected on each distinct population in order to produce accurate models.
Additionally, there is the issue of spontaneous versus non-spontaneous speech. Most data collected is from non-spontaneous speech since it is generally easier to collect. However, spontaneous speech is what is typically of interest in most learning environments.
More work needs to be done in collecting, annotating, and releasing data sets of children’s spontaneous speech in order to help advance this area of research.
Some well-known issues regarding using speech technologies in educational settings (especially with younger learners) include:
- Error rates are high for automatic speech recognition (ASR), especially for children
Naturalistic/spontaneous speech is less studied (and this is typically what occurs in classroom contexts) - Multiple speakers (especially if they move around a space)
- Acoustics in classrooms are challenging (including issue of background noise)
Ethics
Student speech data, like all student data collected, should be gathered, analyzed, and stored in ways that will protect student privacy. Unlike other student data sources, such as assessment responses or clicks on a website, speech can be collected in way that is not transparent or obvious to the student. The concept of having devices all around you that are constantly listening is potentially problematic for creating welcoming and non-invasive learning environments. The ethics of using students’ speech data in a learning context is an important issue that needs to be dissected and discussed in more detail as the situations in which that data will be collected is more defined and understood. The privacy and ethical concerns of using student speech data, either simply for research purposes or as part of a learning system or pedagogical approach, should be a constant thread in all work in this area. As with all data collection, students should be aware of what data is being collected, how it is being collected, and in what ways it is being used. These issues are an integral part of understanding the use of this type of data, not something that is just an afterthought at the end of a project.
Projects
Examples of NSF Cyberlearning projects that overlap with topics discussed in this primer.
More posts: speech-recognition
Other relevant cyberlearning-themed projects:
Speech-Based Learning Analytics for Collaboration (PI: Cynthia D’Angelo).
Formative Assessment with Computational Technologies (FACT) (PI: Kurt VanLehn)
Resources
CIRCL Webinar: Exploring the Promise of Speech Technology for Education Research (January 2017)
Some available tools and datasets:
- COVAREP – Cooperative Voice Analysis Repository for Speech Technologies
- Virtual Human Toolkit – automatic behavior tracking
- SBLAC collaborative learning dataset of middle school student speech (groups of three students working on math problems) [see paper describing dataset] (This dataset will be released in Fall 2017 through the Linguistic Data Consortium.)
Science article: Advances in natural language processing
Multi-modal Learning Data Collection at (Small) Scale blog post by Cynthia D’Angelo on multimodal data collection challenges in classrooms
Readings
Akbacak, M., Burget, L., Wang, W., & Van Hout, J. (2013). Rich system combination for keyword spotting in noisy and acoustically heterogeneous audio streams. In Acoustics, Speech and Signal Processing (ICASSP), 2013 IEEE International Conference on Speech and Signal Processing (pp. 8267–8271).
Barnes, C. P. (1983). Questioning in college classrooms. Studies of College Teaching: Experimental Results, Theoretical Interpretations, and New Perspectives, 61–81.
Bou-Ghazale, S. E., & Hansen, J. H. L. (2000). A comparative study of traditional and newly proposed features for recognition of speech under stress. IEEE Transactions on Speech and Audio Processing, 8(4), 429–442.
Boyd, M., & Rubin, D. (2006). How contingent questioning promotes extended student talk: A function of display questions. Journal of Literacy Research, 38(2), 141–169. doi:10.1207/s15548430jlr3802_2
Bricker, L., Tanimoto, S., & Hunt, E. (1998). Effects of cooperative interactions on verbal communication. In ACM CHI. Pittsburgh, PA.
Cazden, C. B., & Beck, S. W. (2003). Classroom discourse. Handbook of Discourse Processes, 165–197.
Chi, M. T. H. (2009). Active-constructive-interactive: A conceptual framework for differentiating learning activities. Topics in Cognitive Science, 1(1), 73–105. Retrieved from http://www.ncbi.nlm.nih.gov/pubmed/25164801
Erduran, S., Simon, S., & Osborne, J. (2004). TAPping into argumentation: Developments in the application of Toulmin’s Argument Pattern for studying science discourse. Science Education, 88(6), 915–933. Retrieved from http://doi.wiley.com/10.1002/sce.20012
Forbes-Riley, K., & Litman, D. (2005). Correlating Student Acoustic-Prosodic Profiles with Student Learning in Spoken Tutoring Dialogues. In INTERSPEECH (pp. 8–11).
Fredricks, J., McColskey, W., Meli, J., Mordica, J., Montrosse, B., & Mooney, K. (2011). Measuring student engagement in upper elementary through high school: a description of 21 instruments. Issues and Answers Report, 098, 26–27. Retrieved from http://ies.ed.gov/ncee/edlabs
Ford, C. E., & Couper-Kuhlen, E. (2004). Conversation and phonetics: Essential connections. Sound Patterns in Interaction. Cross-Linguistic Studies from Conversation. Amsterdam: Benjamins, 3–25.
Gupta, R., Audhkhasi, K., Lee, S., & Narayanan, S. (2013). Paralinguistic event detection from speech using probabilistic time-series smoothing and masking. In INTERSPEECH (pp. 173– 177).
Hasan, T., Boril, H., Sangwan, A., & Hansen, J. H. L. (2013). Multi-modal highlight generation for sports videos using an information-theoretic excitability measure. In ICASSP.
Herrlitz-Biró, L., Elbers, E., & de Haan, M. (2013). Key words and the analysis of exploratory talk. European Journal of Psychology of Education, 28(4), 1397–1415. Retrieved from http://link.springer.com/10.1007/s10212-013-0172-7
Hymel, S., Zinck, B., & Ditner, E. (1993). Cooperation versus competition in the classroom. Exceptionality Education Canada, 3(1-2), 103–128.
Kaushik, L., Sangwan, A., & Hansen, J. H. L. (2013a). Automatic sentiment extraction from YouTube videos. In Automatic Speech Recognition and Understanding (ASRU), 2013 IEEE Workshop on (pp. 239–244).
Kaushik, L., Sangwan, A., & Hansen, J. H. L. (2013b). Sentiment Extraction from Natural Audio Streams. In ICASSP.
Kolar, J., Lui, Y., & Shriberg, E. (2010). Speaker adaptation of language and prosodic models for automatic dialog act segmentation of speech. Speech Communication, 52(3), 236–245.
Laskowski, K., & Shriberg, E. (2012). Corpus-independent history compression for stochastic turn-taking models. In 2012 IEEE International Conference on Acoustics, Speech and Signal Processing (ICASSP) (pp. 4937–4940). IEEE. doi:10.1109/ICASSP.2012.6289027
Levin, T., & Long, R. (1981). Effective Instruction. ERIC.
Litman, D. J., & Forbes-Riley, K. (2004). Annotating Student Emotional States in Spoken Tutoring Dialogues. In In Proc. 5th SIGdial Workshop on Discourse and Dialogue (pp. 144–153). doi:10.1.1.131.904
Mandal, A., van Hout, J., Tam, Y.-C., Mitra, V., Lei, Y., Zheng, J., … Kathol, A. (2013). Strategies for high accuracy keyword detection in noisy channels. In INTERSPEECH (pp. 15–19).
McNeill, K. L., Lizotte, D. J., Krajcik, J., & Marx, R. W. (2006). Supporting students’ construction of scientific explanations by fading scaffolds in instructional materials. The Journal of the Learning Sciences, 15(2), 153–191.
Mercer, N., Dawes, L., Wegerif, R., & Sams, C. (2004). Reasoning as a scientist: Ways of helping children to use language to learn science. British Educational Research Journal, 30(3), 359–377.
Mercer, N., & Littleton, K. (2007). Dialogue and the development of children’s thinking: A sociocultural approach. Routledge.
Sacks, H., Schegloff, E. A., & Jefferson, G. (1974). A simplest systematics for the organization of turn-taking for conversation. Language, 696–735.
Sangwan, A., & Hansen, J. H. L. (2010). Keyword recognition with phone confusion networks and phonological features based keyword threshold detection. In Signals, Systems and Computers (ASILOMAR), 2010 Conference Record of the Forty Fourth Asilomar Conference on (pp. 711–715).
Shokouhi, N., Ziaei, A., Sangwan, A., & Hansen, J. H. L. (2015). Robust Overlapped Speech Detection and its Application in Word Count Estimation for Prof-Life-Log Data. In International Conference on Acoustics, Speech and Signal Processing (ICASSP).
Slavin, R. E. (1991). Synthesis of research of cooperative learning. Educational Leadership, 48(5), 71–82.
Tobin, K. (1987). The role of wait time in higher cognitive level learning. Review of Educational Research, 57(1), 69–95. doi:10.3102/00346543057001069
Van Zee, E. H., Iwasyk, M., Kurose, A., Simpson, D., & Wild, J. (2001). Student and teacher questioning during conversations about science. Journal of Research in Science Teaching, 38(2), 159–190. doi:10.1002/1098-2736(200102)38:2<159::AID-TEA1002>3.0.CO;2-J
West, R., & Pearson, J. C. (1994). Antecedent and consequent conditions of student questioning: An analysis of classroom discourse across the university. Communication Education, 43(4), 299–311.
Wrede, B., & Shriberg, E. (2003). Spotting “hotspots” in meetings: Human judgments and prosodic cues. In Proceedings of Eurospeech 2003 (pp. 2805–2808). Geneva.
Zhou, G., Hansen, J. H. L., & Kaiser, J. F. (2001). Nonlinear feature based classification of speech under stress. Speech and Audio Processing, IEEE Transactions on, 9(3), 201–216.
Ziaei, A., Sangwan, A., & Hansen, J. H. L. (2014). A speech system for estimating daily word counts. In Interspeech 2014.
Citation
Primers are developed by small teams of volunteers and licensed under a Creative Commons Attribution 4.0 International License. After citing this primer in your text, consider adding: “Used under a Creative Commons Attribution 4.0 International License (http://creativecommons.org/licenses/by/4.0/).”
Suggested citation:
D’Angelo, C., & Dorsey, C. (2017). CIRCL Primer: Speech Technology and Learning. In CIRCL Primer Series. Retrieved from http://circlcenter.org/speech-technology-and-learning