An interview with Eli Meir about his NSF-funded project to use dynamic formative assessment models to enhance learning of the experimental process in biology.
What is the big idea of your project? What is the problem you’re trying to solve?
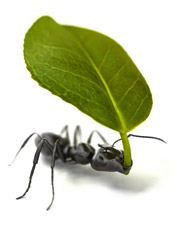
We make virtual labs that have some very open-ended components that let students explore and discover concepts on their own. These components are trying to address higher order thinking skills and big ideas. It is hard to assess whether students are understanding those skills with standard auto-gradable assessments like multiple choice. While you can assess higher-order thinking skills with more open-ended assessments like asking students to write an essay, or draw pictures, or make graphs, and so on, those are not easily auto-gradable. So if you want to do those kinds of things in a large class, grading is hard for many professors. Furthermore, you can’t give students immediate feedback on those types of assessments – the feedback only comes a week later after the professor has had time to do the grading, which is too late for most of the learning that comes from more immediate feedback.
NSF Project Information
Title: DIP: Using dynamic formative assessment models to enhance learning of the experimental process in biology
Award Details
Investigators: Eli Meir, Joel Abraham, Eric Klopfer, Zhushan Li
Web site: Simbiotic Software
In our grant, we are focusing on giving students immediate feedback on open-ended, higher-order thinking skill tasks, by trying to take the kind of assessments we’d like to do–– more open ended assessments––and putting enough constraints on those to be able to have a computer algorithm recognize what the students is thinking and give them appropriate feedback. We’ve specifically been focusing on what we are calling intermediate constraint questions that where students can still construct their own answers, but within certain boundaries that make analysis easier. For example we’ve created two different interfaces that can replace a short-answer essay question, which we call LabLibs (modeled after the madlibs game) and WordBytes (modeled after fridge poetry sets). We’ve also created constrained graphing exercises and a constrained simulation-based experimental design exercise. In the latter, when students design experiments we can recognize, for instance, when they haven’t included a control or when they’re varying more than one variable at a time.
My impressions is that the field hasn’t thought very much about constraints; they’ve been enchanted by algorithms and data, and the idea that if you get enough data and get enough computers and have smart enough machine learning, you’ll be able to figure everything out. Instead, we’re focusing on constraints to make the machine’s job easier; it’s kind of low-hanging fruit in a way. And in this process, we’re improving our SimBio Virtual Labs to include feedback in places where we weren’t previously able to.
Can you say more about the research you are doing?
Quick Facts
Age: Primarily undergraduate; some high school
Subject area: Biology
Setting: Face to face, in person; remotely, online, at a distance
Geographic location: Colleges and universities across North America
We’re doing a lot of validation around questions such as: How much does putting constraints on what the student is doing affect what they do? Are we actually capturing what the student is thinking? There is design research as well, about how to make our interfaces so that they are constrained and still allow the students to do interesting things.
The last year of the project will be devoted to more summative assessment which addresses whether giving student feedback actually helps students learn these concepts. The software will be used in several classes, and we’ll do pre- and post testing to assess learning gains, compare pre- and post tests across classes with and without feedback, and then culminate with split class studies––where half of the students are working with the constrained interfaces and the other half are doing something else––and look for a difference in learning gains.
Can you give an example of a constraint, and how you’d compare across classes?
One interface that we’re building, called WordBytes, is modeled after fridge poetry sets. We give students sets of words and phrases which they have to use them to construct sentences to answer a question. We’ve come up with algorithms that allow us to give students quite fine-grained feedback depending on the answer they compose, and the feedback comes right away. This has advantages over both multiple choice and short-answer essay questions. In multiple choice, the student also gets feedback right away, but the thought process in coming to the answer is much lower-order. In essay questions, they construct their own answer, but usually get no feedback (or if there is feedback, it’s not as fine-grained).
A big advantage when they get immediate feedback is they can then go through a learning process with their answer. Students will often that will start off with a wrong answer, and then get the feedback, and then after 2 or 3 tries, end up with the correct answer. So that’s indirect evidence of learning — given that you could make millions of combinations out of the words and phrases we’re giving them, the fact that they can come to a correct answeris evidence that they are actually figuring out what their mistake was. It’s not just picking the next answer out of 4 multiple-choice answers.
What is the learner experience like? If I were a student participating in this project, what would I experience?
Our labs are used on about a fifth of campuses in North America. Students get a virtual lab that includes one or more fairly sophisticated simulations of some biological system. They play with those simulations by changing parameters or by doing an experiment like a researcher would. Unlike many virtual biology labs, we make our tools quite flexible so that you get the same conceptual experience as in a wet lab. For instance, we might let students add or remove critters in an ecosystem, and they decide how many to add, where to add them, how long to run the simulation, and which critters to measure afterwards to get their results.
Students in this study are doing these virtual labs very similarly to how other students would be doing SimBio labs, but embedded in the labs are the intermediate-constraint interfaces that we’re developing. For the most part, the students don’t know they are doing anything different or nonstandard. They are just going along and encounter questions they have to answer, in a slightly different interface, or they encounter a simulation they have to play with, but they don’t realize they’re only getting certain tools and not as many as they might have got a year before. Then they get feedback and can change what they do based on the feedback.
What is the teacher’s role and what is their experience?
Some of the teachers have used the software in the past, and some are brand new to it. In the study condition, the biggest thing that will change for them is that they have to do less grading if there are no short answer questions, so it saves them time. They also get a little more information about their students because the system reports out what their students have been doing in these interfaces. In general, it is not a lot of extra work for the professors although there is some coordination involved when we ask them to do pre and post tests.
You have partners at universities; what is their role on the project?
It’s been a really productive partnership. Our MIT partners are contributing a lot to the design ideas, and to assessing the innovation––how to built the pre- and post tests and analyze that data. Our partners at Cal State are contributing to the design ideas, and will be contributing to the summative assessments at the end. Our partners at Boston College are focused on statistical analysis of the data. We (SimBio) are a company rather than an academic lab, and within the company we also have multidisciplinarity in that we have an education person, biologists, people who teach, programmers, etc. So in that sense, we built our company to try to do what cyberlearning is trying to encourage. But that said, partnering has been great, and the project is a lot stronger because of the contributions of the partners.
How do you see your project advancing the broader cyberlearning field?
I think the things we’ll contribute for the larger field are some demonstrations of interfaces that fit this idea of using constraints at scale. We’ll show interfaces that work at scale. We’ll also have some information to contribute on algorithms to analyze those kind of constrained but still fairly open-ended data. And probably one of our biggest contributions will be rules of thumb for how to use these interfaces and make them work. We’re trying them in different places and have failures as well as successes. So by the end we should have something along the lines of “if you want to use this type of constrained presentation, here are the top 10 things to pay attention to in order to maximize the chance of success.”